The Essential Role of Data Governance in AI Development
May-13-2024
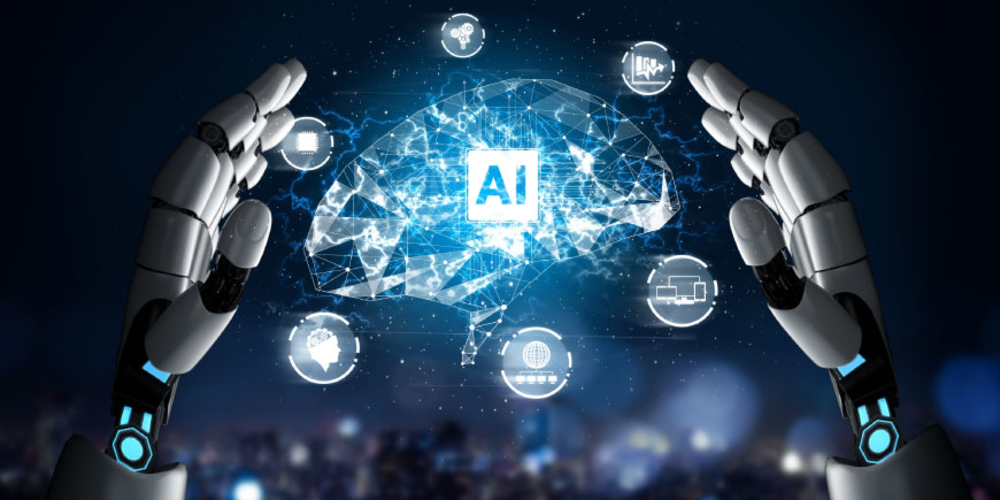
In the rapidly evolving landscape of artificial intelligence (AI) and machine learning (ML), the spotlight often falls on the innovative engineers who design and optimize these technologies. However, an equally crucial player in ensuring the success and ethical deployment of AI models is the data governance architect. Satish Jayanthi, CTO & co-founder of Coalesce, underscores the foundational role of data governance in the realm of AI, emphasizing its critical importance in the integrity and value extraction from AI and ML models.
The Foundation of AI Success
While the allure of designing AI systems often gravitates towards the creativity and technical prowess of prompt engineers, Jayanthi points out that the quality and integrity of the data used are what truly determine the outcomes of these models. The role of a data governance architect, therefore, becomes indispensable in laying the groundwork for effective AI initiatives. This involves meticulous pre-processing and standardization of data to ensure that AI models are built upon a robust and reliable dataset. Without this foundational step, even the most advanced AI systems can falter, hampered by flawed or biased data inputs.
Challenges in Establishing Effective Data Governance
The path to establishing effective data governance is fraught with challenges. Jayanthi highlights the cultural and technical hurdles that organizations face in this endeavor. Cultivating an organizational culture that values and understands the importance of data governance is a significant challenge. This holistic approach requires cooperation across various departments, transcending traditional IT roles. Moreover, the complexity and diversity of data sources add another layer of difficulty in standardizing data governance practices. Staying abreast of the swift progress in AI and ML technologies requires a flexible and evolving governance approach to guarantee the security and integrity of data.
The Impact of Poor Data Governance
Inadequate data governance can severely compromise the outcomes of AI and ML projects. Jayanthi stresses that compromised data integrity leads to biased or inaccurate AI outputs, posing serious ethical and operational risks, especially in critical sectors. The lack of standardized data quality checks can result in inconsistent AI/ML model performances, undermining the reliability and scalability of AI solutions. As AI and ML technologies increasingly permeate various aspects of society, ensuring data accuracy and mitigating biases become not just technical challenges but ethical imperatives.
The Principle of 'Garbage In, Garbage Out'
The adage 'garbage in, garbage out' is particularly pertinent in the context of AI model training. Jayanthi elaborates on how the quality of input data directly influences the fidelity of AI outputs. Flawed input data, characterized by inaccuracies, biases, or incompleteness, leads AI models to replicate these deficiencies in their outputs. This principle underscores the importance of rigorous data governance in ensuring the accuracy and fairness of AI models, exemplified by the challenges faced in applications like facial recognition from diverse datasets.
Emerging Trends and Future Directions
Looking ahead, Jayanthi identifies several key trends in data governance that companies need to be aware of. The rise of automated tools for data governance, the increasing emphasis on ethical AI, and the shift towards cloud-based data solutions are reshaping the landscape of data governance. These trends highlight the need for robust governance frameworks that can adapt to the complexities of distributed systems and evolving technological paradigms. The focus on ethical AI also signals a broader regulatory and societal push towards ensuring AI technologies are deployed responsibly and equitably.
Strategies for Enhanced Data Governance
To navigate the complexities of data governance in preparation for AI and ML adoption, Jayanthi recommends a multi-faceted approach. Developing comprehensive data governance policies is a critical first step, establishing clear standards for data handling and alignment with AI and ML objectives. Cultivating a data-conscious culture within the organization is equally important, fostering a shared responsibility for data integrity. Continuous evaluation and refinement of governance strategies are essential to adapt to the evolving data and technology landscape, ensuring that organizations remain at the forefront of AI and ML innovation.
In conclusion, the role of data governance in the development and deployment of AI and ML technologies is of paramount importance. As organizations navigate the complexities of extracting value from AI and ML models, the insights and strategies outlined by Satish Jayanthi serve as a valuable guide. By prioritizing robust data governance, companies can ensure the integrity, reliability, and ethical deployment of AI systems, paving the way for transformative innovations across industries.